Efficiency and innovation are essential for success, driving businesses to adopt AI solutions that simplify processes, enhance accuracy, and enable smarter decisions. With the AI market projected to reach $184 billion globally by 2024 and studies showing a 40% potential boost in employee productivity by 2035, the impact of AI is undeniable.
However, not all platforms are created equal, making it critical to understand the core features that set them apart. From predictive analytics to automated control systems, selecting the right capabilities can transform strategies and unlock new levels of operational excellence.
This article provides a clear, informative guide on AI technologies, their real-world applications, how to select the right AI solution, and strategies to maximize their value.
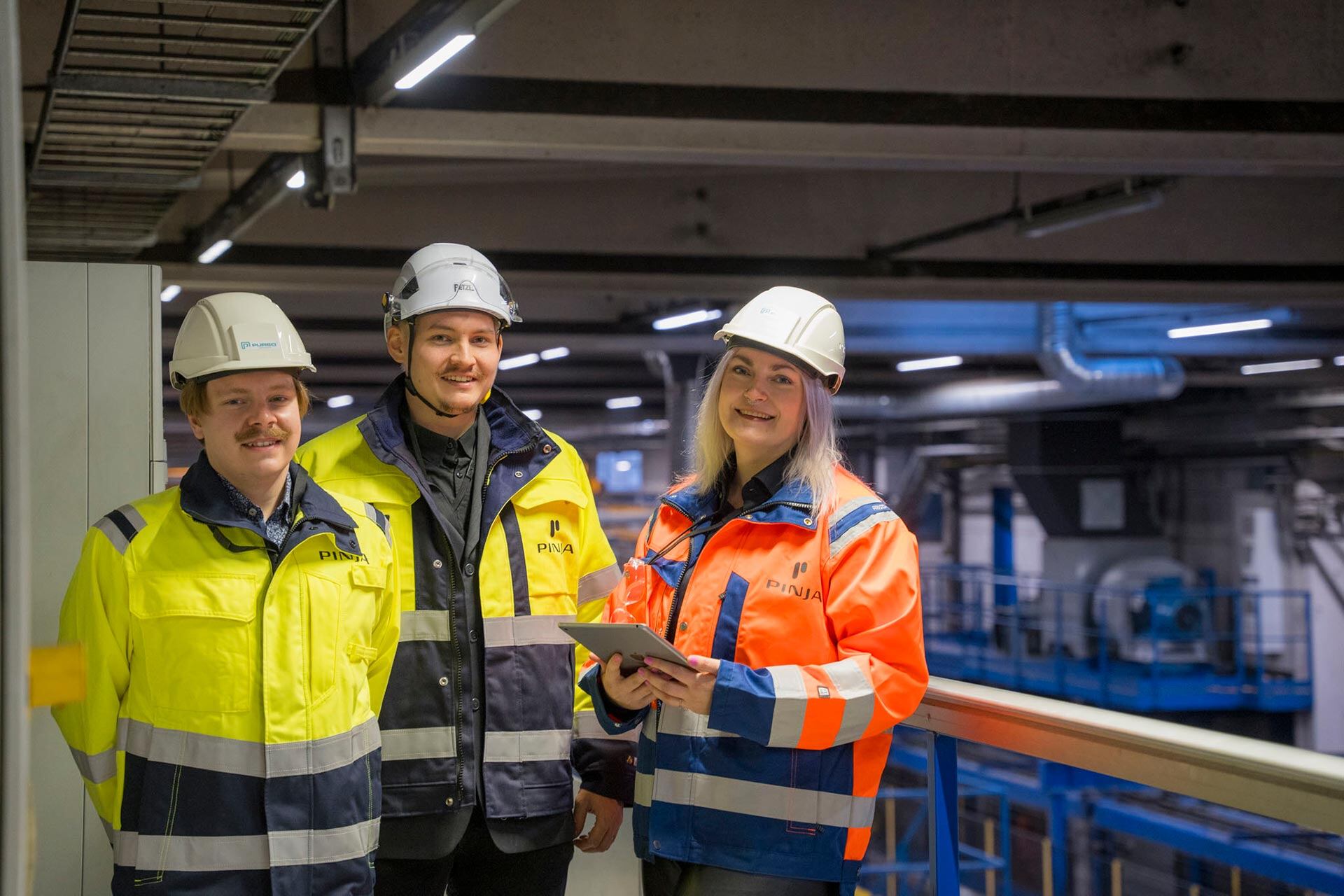
Breaking Down AI and How It Powers Business Operations
AI represents a collection of technologies designed to simulate human intelligence. These systems analyze data, detect patterns, make predictions, and automate processes, offering businesses the ability to optimize efficiency, minimize human error, and generate actionable insights.
Key technologies underpinning AI solutions include:
-
Machine learning (ML), focuses on developing algorithms that learn from both historical and real-time data. This allows businesses to forecast trends, improve workflows, and identify patterns that might otherwise go unnoticed.
-
Deep learning, a subset of ML, utilizes complex neural networks to process large datasets. It excels in solving advanced problems, such as image recognition, speech processing, and nuanced decision-making tasks.
-
Autonomous systems, powered by AI, operate with minimal human intervention. These systems improve efficiency across repetitive or resource-intensive workflows, such as manufacturing, logistics, and supply chain management.
Another key aspect of AI is its ability to process language and adapt to dynamic tasks. Foundation models, large-scale AI systems pre-trained on vast datasets, can be fine-tuned for specific applications such as content creation, data categorization, or anomaly detection.
Complementing this is natural language processing (NLP), which enables machines to interpret, understand, and respond to human language. NLP is widely employed for automating customer communication, generating reports, and analyzing unstructured textual data.
When businesses face complex optimization challenges, traditional methods often fall short. Metaheuristics, a class of AI-driven algorithms, provide a solution by efficiently tackling large-scale, dynamic problems. These algorithms improve resource allocation, streamline production planning, and optimize decision-making processes.
By rapidly identifying optimal or near-optimal solutions, they improve workflows, logistics, and operational strategies in industries ranging from manufacturing to transportation.
AI technologies transform data into actionable outcomes, enabling businesses to improve efficiency and decision-making.
Real-World Applications of AI Across Industries
1. Optimization and Efficiency Enhancement
One significant area where AI contributes is workflow optimization. By analyzing operational data, AI systems can identify bottlenecks that slow processes down and propose solutions to remove them. Automating repetitive tasks, such as data entry or routine inspections, allows human workers to focus on higher-value activities, further improving efficiency.
In addition, AI can allocate resources dynamically, ensuring machines, materials, and labor are utilized optimally. This results in reduced downtime, smoother operations, and measurable productivity improvements.
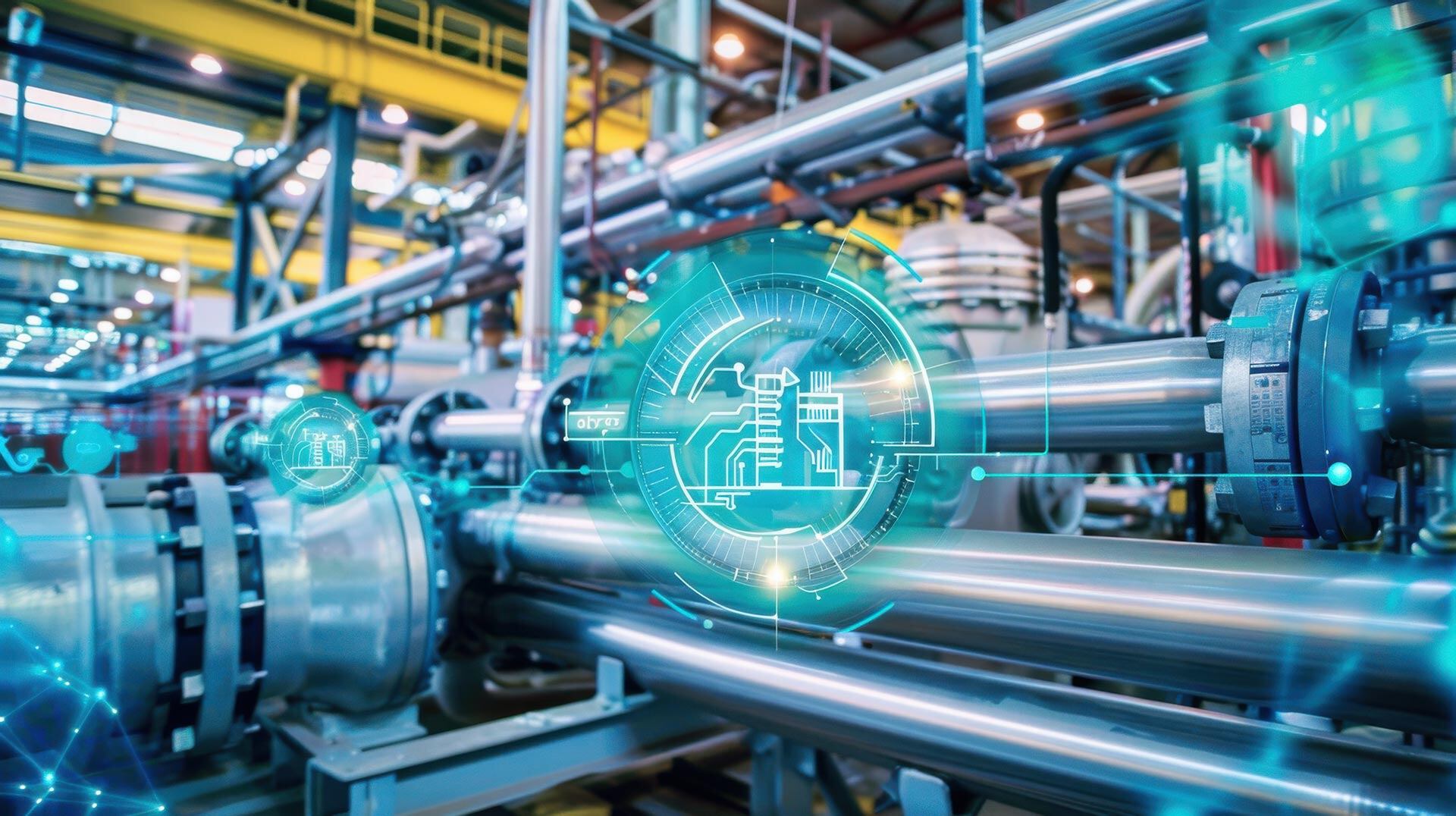
Another key application is in supply chain management. AI helps businesses forecast demand more accurately, reducing the risks of overstocking or running out of inventory. This predictive capability ensures that inventory levels are maintained at an optimal balance, improving cost efficiency.
Moreover, logistics operations benefit from AI-driven route planning, which minimizes transport distances and fuel consumption. Companies can save significantly on transportation costs while ensuring faster delivery times.
Energy optimization is another critical domain where AI is making a difference. By analyzing energy consumption patterns across production facilities, AI identifies inefficiencies and recommends actionable changes.
For example, adjusting machine schedules to off-peak hours or implementing predictive maintenance can lower energy bills. AI models can also predict energy demand fluctuations, allowing facilities to align their usage with available supply.
AI improves industrial efficiency by automating workflows, optimizing resources, and improving energy usage.
2. Predictive Analytics and Reporting
Predictive analytics and reporting are essential in AI-driven solutions, enabling organizations to optimize processes, reduce risks, and make informed decisions. A key application is maintenance forecasting, where AI analyzes historical and real-time data to predict equipment failures, allowing for proactive maintenance that minimizes costly disruptions.
This is vital for industries like manufacturing and energy, where reliability is crucial.
AI also generates operational insights by processing large datasets to identify inefficiencies and opportunities. Real-time reporting tools help decision-makers address issues such as process bottlenecks or inventory shortages swiftly.
Additionally, AI transforms knowledge management by centralizing organizational information, enhancing collaboration, and breaking down silos. Tools like Fabric centralize and streamline access to organizational knowledge, making it easier for teams to collaborate and make decisions based on accurate and up-to-date information.
Process automation further drives efficiency by handling repetitive tasks and enabling robotics to perform precision work, such as quality inspections. Lastly, AI supports strategic decisions by analyzing data and simulating scenarios, helping leaders reduce uncertainty and align strategies with long-term goals.
Predictive analytics transforms raw data into actionable insights, driving smarter decisions and operational excellence.
3. Generative and Adaptive AI Applications
Generative and adaptive AI solutions are revolutionizing workflows and customization. AI automates tasks like report generation, data categorization, and content creation, significantly reducing time spent on manual processes while maintaining consistency and accuracy. This streamlining enhances productivity across content management systems.
Adaptive AI brings personalization to the forefront, using customer data and feedback to tailor products and services. From fine-tuning configurations to rapid prototyping through generative design models, businesses can respond to unique client needs with agility.
AI-based document intelligence modules further boost efficiency by quickly analyzing organizational databases to retrieve relevant information, enabling informed decision-making and reducing delays.
Service chatbots add another layer of efficiency, automating real-time interactions both for external clients and internal operations. These tools not only improve response times but also integrate seamlessly into workflows, delivering support where and when it’s needed most.
Generative and adaptive AI redefines efficiency by merging automation with intelligence.
4. Diagnostics and Quality Control
AI-driven diagnostics and quality control are reshaping industrial processes by enhancing precision, reliability, and efficiency.
Anomaly detection is a key application, where AI-powered systems monitor production in real time to identify irregularities. For example, if a machine's performance deviates from expected parameters, the system flags the issue immediately, preventing defects and minimizing waste.
By continuously analyzing data patterns, AI detects subtle shifts that human operators might miss, ensuring smoother operations and reduced downtime.
Quality assurance further benefits from AI by automating inspections traditionally performed manually. Using computer vision and machine learning, AI evaluates products against industry standards with unmatched speed and accuracy, inspecting hundreds of items per minute while eliminating variability. This ensures consistent product quality and streamlines processes.
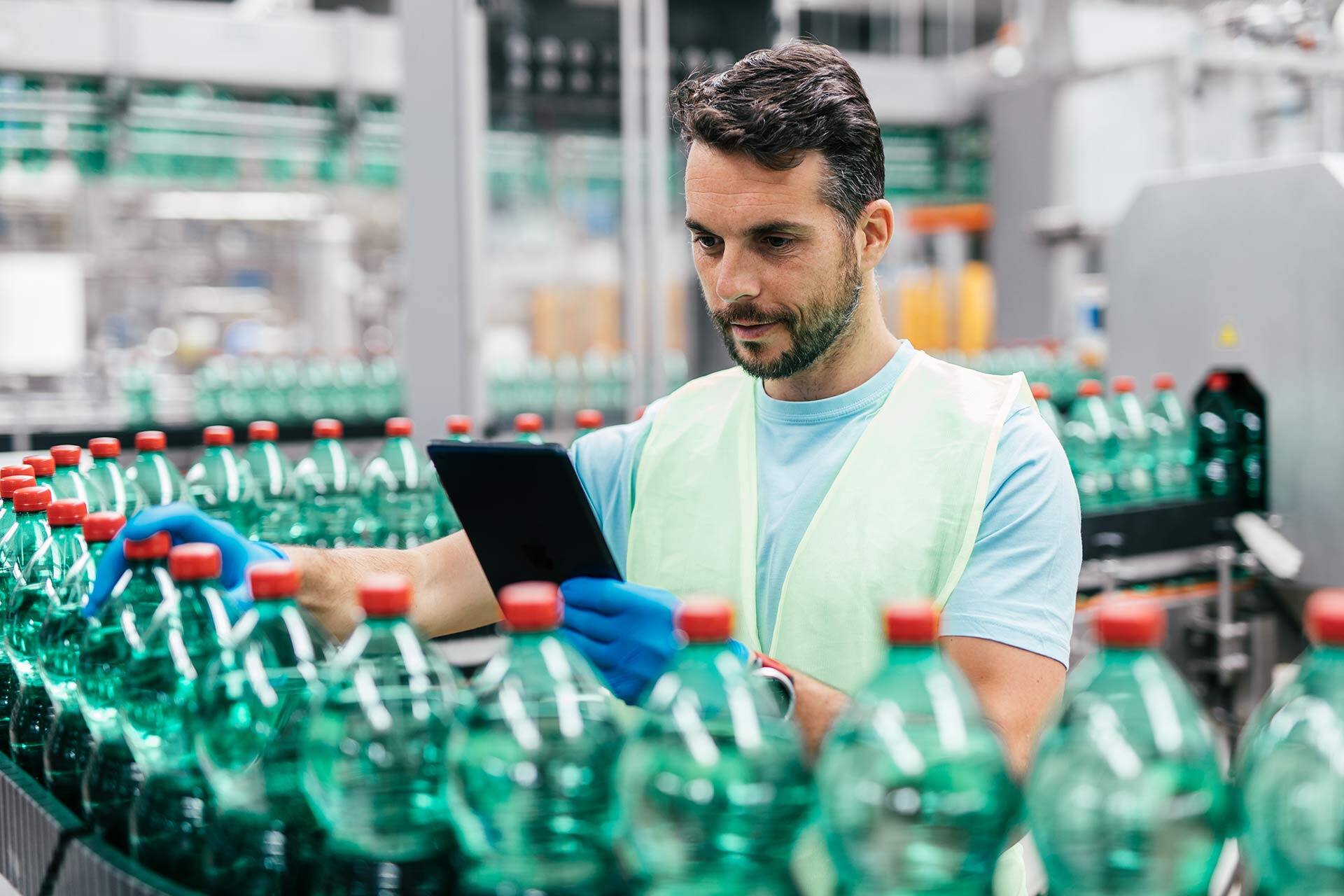
Automated quality checks ensure consistency and reduce human error in industrial processes.
A Practical Guide to Selecting the Right AI Solution
Step 1: Define Business Objectives and AI Requirements
Defining business objectives and AI requirements ensures initiatives are aligned with strategic goals, addressing specific challenges and delivering measurable outcomes.
To start, AI goals must connect directly to overarching business objectives, such as improving operational efficiency, enhancing product quality, or enabling data-driven decision-making. This alignment positions AI as a tool for long-term growth and competitive advantage, rather than a standalone effort.
Identifying pain points is equally critical. Businesses should evaluate challenges like production bottlenecks, resource inefficiencies, or high costs to pinpoint areas where AI can provide the most value. For instance, automating repetitive tasks can reduce labor expenses in manufacturing, while optimizing routes can save time and fuel in logistics.
Establishing clear success metrics, such as reducing downtime or achieving specific cost savings, ensures AI investments are both measurable and impactful, driving meaningful organizational improvements.
Clear objectives and metrics ensure that AI implementation drives measurable and strategic value.
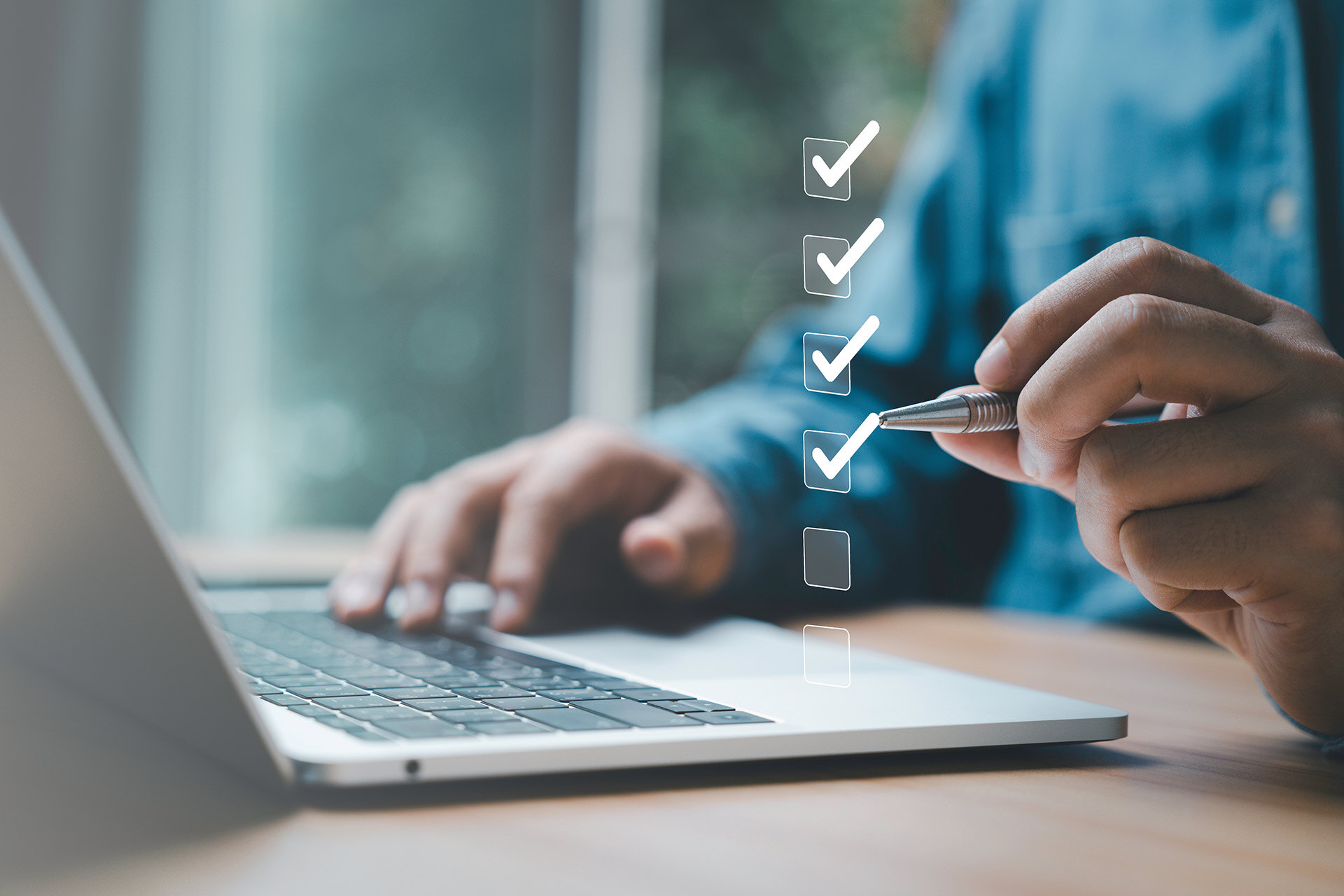
Step 2: Assess Business Readiness for AI
Implementing AI solutions for industrial process optimization requires businesses to first assess their readiness. This involves evaluating infrastructure, data resources, and workforce capabilities to ensure a strong foundation for successful AI integration.
Assessing infrastructure is a key step. Businesses must determine if their hardware, software, and networks can handle AI’s computational demands, such as those required by machine learning algorithms. If gaps exist, upgrades like cloud platforms or high-performance servers may be necessary.
High-quality data is equally essential. AI systems depend on large, accurate datasets for training and operation. Organizations should evaluate the completeness, relevance, and reliability of their data. If data quality is lacking, improvements like enhanced data collection and validation should be prioritized.
Workforce capabilities are another critical factor. Adopting AI often requires specialized expertise. Conducting a skills gap analysis helps identify training needs or hiring requirements, ensuring teams are prepared for AI implementation.
A successful AI implementation begins with an honest assessment of infrastructure, data, and workforce readiness.
Step 3: Evaluate AI Solution Capabilities
Evaluating the capabilities of an AI solution is crucial to ensure it aligns with business needs and supports long-term growth. The right solution should address current challenges while adapting to future demands, avoiding costly mismatches and integration issues.
Scalability and flexibility are key considerations. As businesses grow and operations become more complex, the AI solution must scale seamlessly, whether by managing larger datasets or accommodating expanded workflows. Flexibility is equally vital, enabling the system to adapt to evolving technology and industry shifts, ensuring it remains relevant over time.
Compatibility with existing systems is another critical factor. AI should integrate effortlessly with tools like ERP and MES systems to maintain smooth operations and efficient data flow. Poor integration can create bottlenecks and inefficiencies, undermining the solution’s potential.
Lastly, customization is essential. A tailored AI system can address unique operational requirements, maximizing its value. Standardized solutions often fail to meet the nuanced demands of specific industries, making adaptability a must.
Scalable, compatible, and customizable AI solutions are the foundation of successful implementation in dynamic business environments.
Step 4: Select the Right AI Vendor or Partner
Selecting the right AI vendor or partner can define the success of your process optimization efforts. The vendor’s expertise, security practices, and support services all contribute to the solution’s overall impact, making careful evaluation essential.
A vendor’s industry expertise often separates exceptional partners from average ones. Providers familiar with your operational environment can better navigate specific challenges and design tailored solutions. Additionally, compliance with data security regulations like GDPR or HIPAA is non-negotiable.
Choosing a vendor with robust security measures ensures that sensitive data remains protected and regulatory standards are met.
Strong support systems also indicate a reliable vendor. From onboarding resources to team training, comprehensive assistance ensures smoother adoption. Equally, ongoing maintenance and timely updates are crucial for sustaining performance over time.
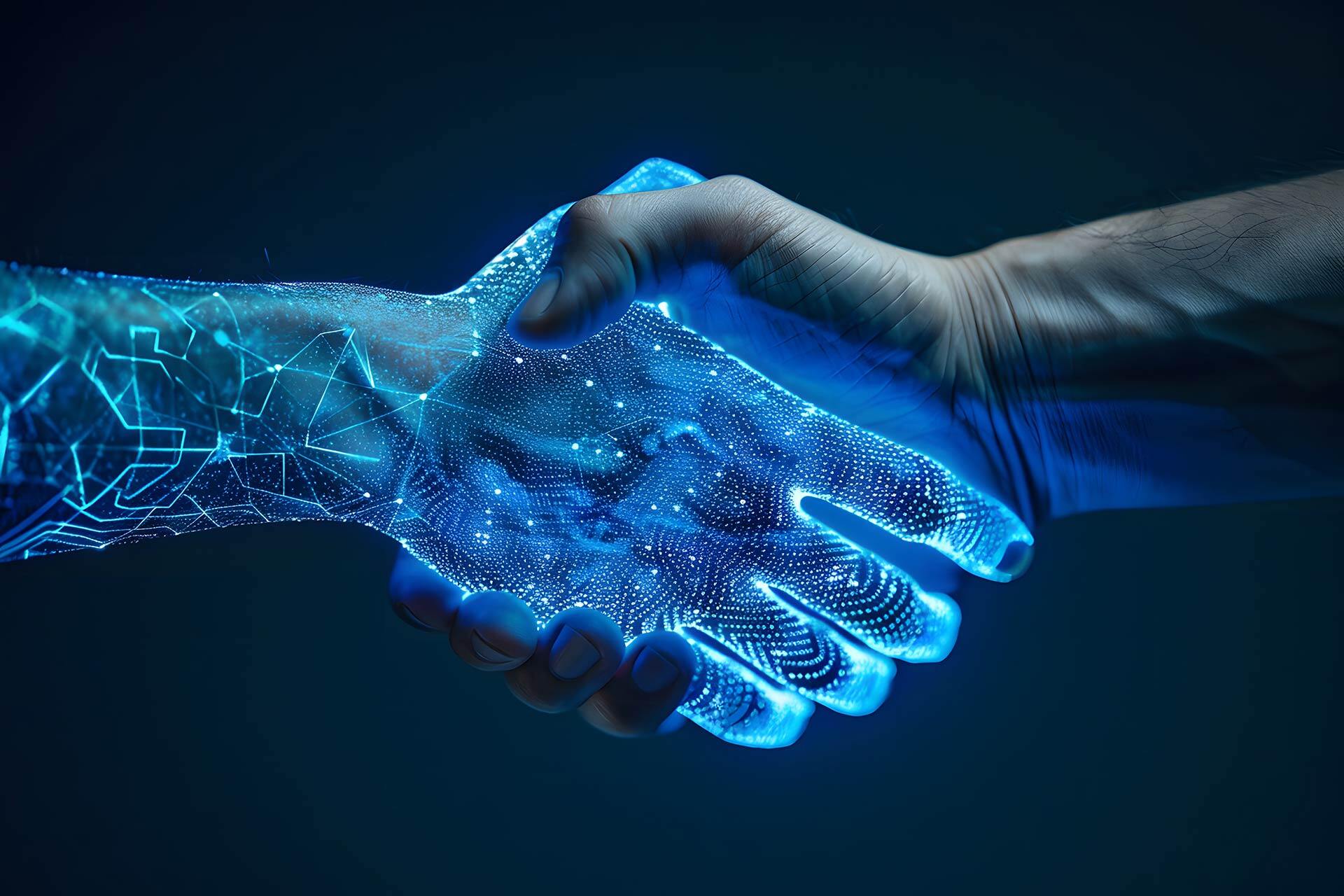
Finally, financial considerations cannot be overlooked. Assess both upfront costs and potential long-term returns to identify the best balance between value and affordability. Comparing multiple vendors on these metrics ensures a well-rounded, strategic choice.
The success of an AI solution hinges on selecting a vendor with both technical expertise and a commitment to long-term support.
The right partner must not only meet key criteria but also deliver value aligned with your goals. Pinja’s AI solutions excel in optimizing efficiency and driving innovation. With tools like the iPES production planning system for automated scheduling and AI-powered analytics for smarter decision-making, Pinja empowers businesses to reduce costs and improve operations.
Our expertise in predictive maintenance, supply chain optimization, and sustainability reporting ensures seamless integration, compliance, and growth. Tailored to diverse needs, our AI solutions customizable solutions transform workflows and deliver lasting ROI.
Step 5: Plan for AI Implementation
Effective planning is the backbone of a successful AI implementation in industrial processes. A clear, phased approach minimizes risks, aligns initiatives with business objectives, and ensures scalable adoption.
Pilot projects are a smart starting point. Small-scale deployments validate specific use cases and provide a controlled environment to test AI functionality. These pilots reveal potential challenges, allowing teams to fine-tune configurations before expanding across the organization.
Addressing issues early can reduce the risk of costly missteps during full-scale deployment.
Once pilot projects demonstrate success, a phased implementation strategy ensures smoother integration. Starting with high-impact processes, such as production scheduling or inventory management, allows organizations to focus on measurable improvements.
Each phase builds confidence and momentum while providing opportunities to resolve unforeseen challenges and refine the system for broader use.
Stakeholder involvement is equally essential. Engaging leadership aligns the project with strategic goals, while collaboration with IT teams ensures technical feasibility. Involving end-users promotes workflow compatibility, reducing resistance and fostering adoption.
A well-planned, collaborative approach turns AI implementation into a seamless, organization-wide success.
Pilot projects and phased strategies reduce risks and build momentum for AI adoption.
Getting the Most Out of Your AI Investment
1. Continuous Improvement Through Feedback and Updates
Continuous improvement is the key to unlocking the full potential of AI solutions in industrial processes. AI systems thrive on refinement, evolving to stay accurate and relevant as operational needs shift. Achieving this requires a dynamic approach that balances user insights with proactive technical updates.
User feedback is an invaluable resource for improving AI performance. Employees interacting with the system daily often uncover nuances — like inefficiencies, overlooked data patterns, or gaps in functionality — that may not be evident otherwise.
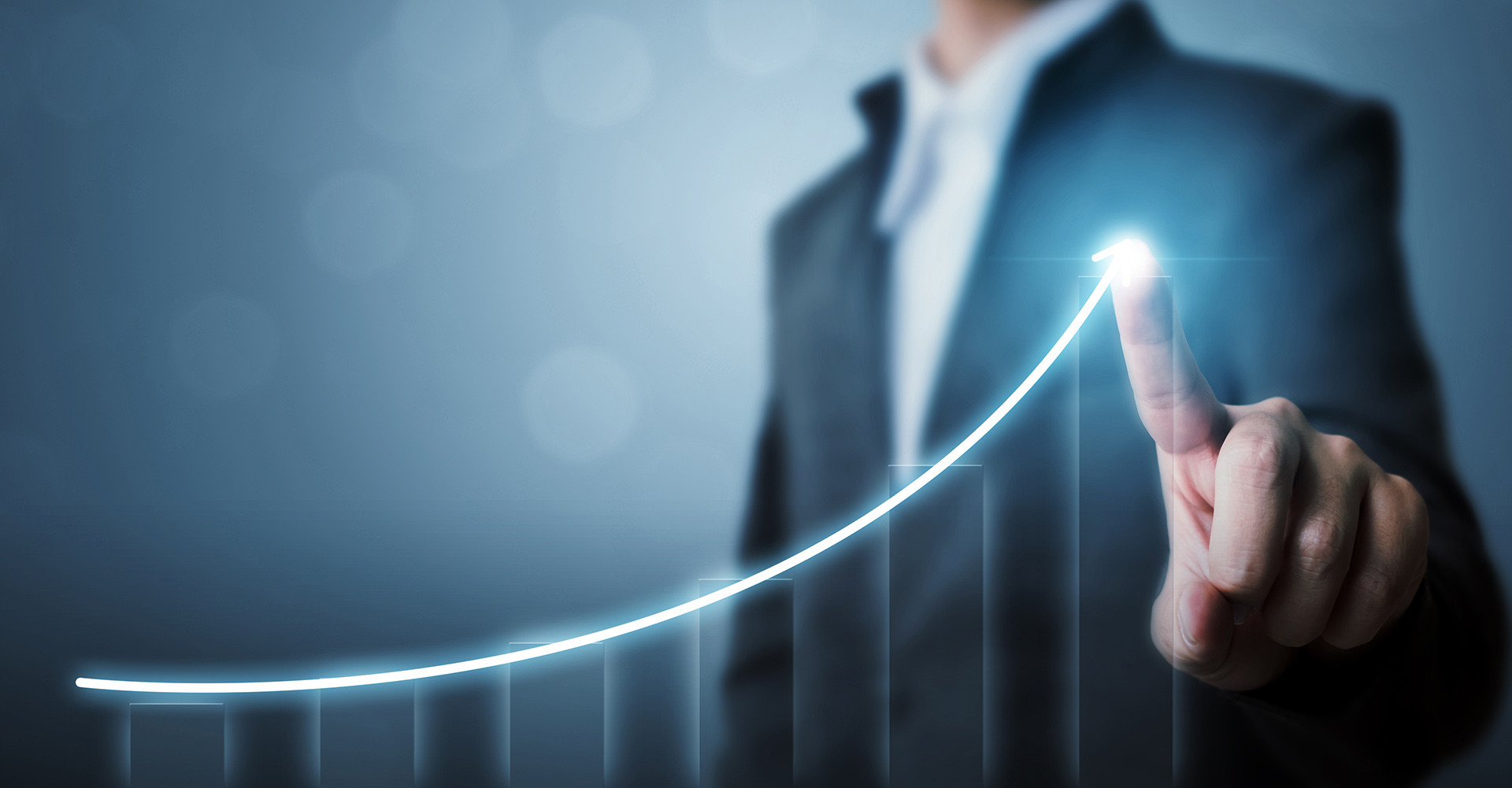
For instance, an AI-driven scheduling tool might falter with specific variables, or predictive maintenance might miss subtle yet critical trends. Leveraging these insights enables businesses to fine-tune workflows, enhance algorithms, and optimize real-world performance.
On the technical side, AI systems must continuously learn. Updating models with fresh, high-quality data ensures predictions remain accurate, while software upgrades introduce new features, fix bugs, and bolster security.
Continuous feedback and updates are the foundation of a successful AI lifecycle.
2. Integration with Existing Systems
Integrating AI solutions with existing systems is crucial for effective industrial process optimization. Without proper integration, data silos and workflow disruptions can diminish AI’s benefits. Ensuring seamless connections with tools like ERP, CRM, or MES requires careful planning.
Interoperability is key—AI must communicate smoothly with current systems, facilitated by APIs and middleware to maintain efficient data flow. For instance, linking AI-driven predictive maintenance with MES ensures actionable insights reach operations instantly.
Aligning AI with workflows prevents disruptions, while shared insights across systems enhance decision-making. Connecting AI supply chain tools with ERP improves inventory management and resource allocation.
Effective integration ensures AI solutions work in harmony with existing systems, driving both efficiency and collaboration.
3. Employee Training for Efficient Utilization
Equipping employees with the skills to use AI tools effectively ensures these technologies deliver their full value in industrial processes. Proper training enables teams to utilize AI as intended, avoiding misapplication or inefficiencies that can hinder outcomes.
A structured approach to upskilling and fostering collaboration bridges the gap between technology and daily workflows.
Training programs should focus on both technical and operational aspects. Employees need to operate AI systems confidently, interpret outputs, and integrate insights into their responsibilities.
For instance, a production manager benefits from understanding predictive maintenance alerts, while a quality control specialist gains value from learning anomaly detection algorithms.
Since technical expertise varies across teams, modular training programs provide tailored instruction, addressing specific roles and needs. Beyond skills, fostering collaboration between employees and AI systems encourages alignment. When teams see AI as a partner that enhances decision-making and problem-solving, they are motivated to explore innovative ways to use it effectively.
Employee training ensures AI tools are utilized efficiently and integrated into workflows effectively.
4. Measuring and Monitoring ROI for Long-Term Success
Measuring and monitoring the ROI of AI solutions ensures they deliver measurable benefits and align with business goals.
Tracking KPIs tied to AI objectives is a vital first step. Metrics such as cost savings, operational efficiency, and error reduction provide concrete insights into performance. For instance, a manufacturing firm might measure reduced downtime, increased production rates, or fewer defects. Regularly comparing these results against benchmarks highlights the value of AI implementations.
Analyzing ROI data enables businesses to pinpoint areas of success and improvement. High cost savings might justify scaling similar solutions, while stagnant efficiency gains could indicate the need for refined algorithms or better training data. Persistent errors may signal gaps in AI capabilities or integration challenges.
This iterative approach ensures AI remains aligned with evolving goals and fosters smarter resource allocation for future projects. Data-driven insights empower your organization to prioritize investments that promise the highest returns, sustaining growth and competitiveness.
Monitoring AI performance through KPIs and refining strategies based on results ensures sustainable ROI.
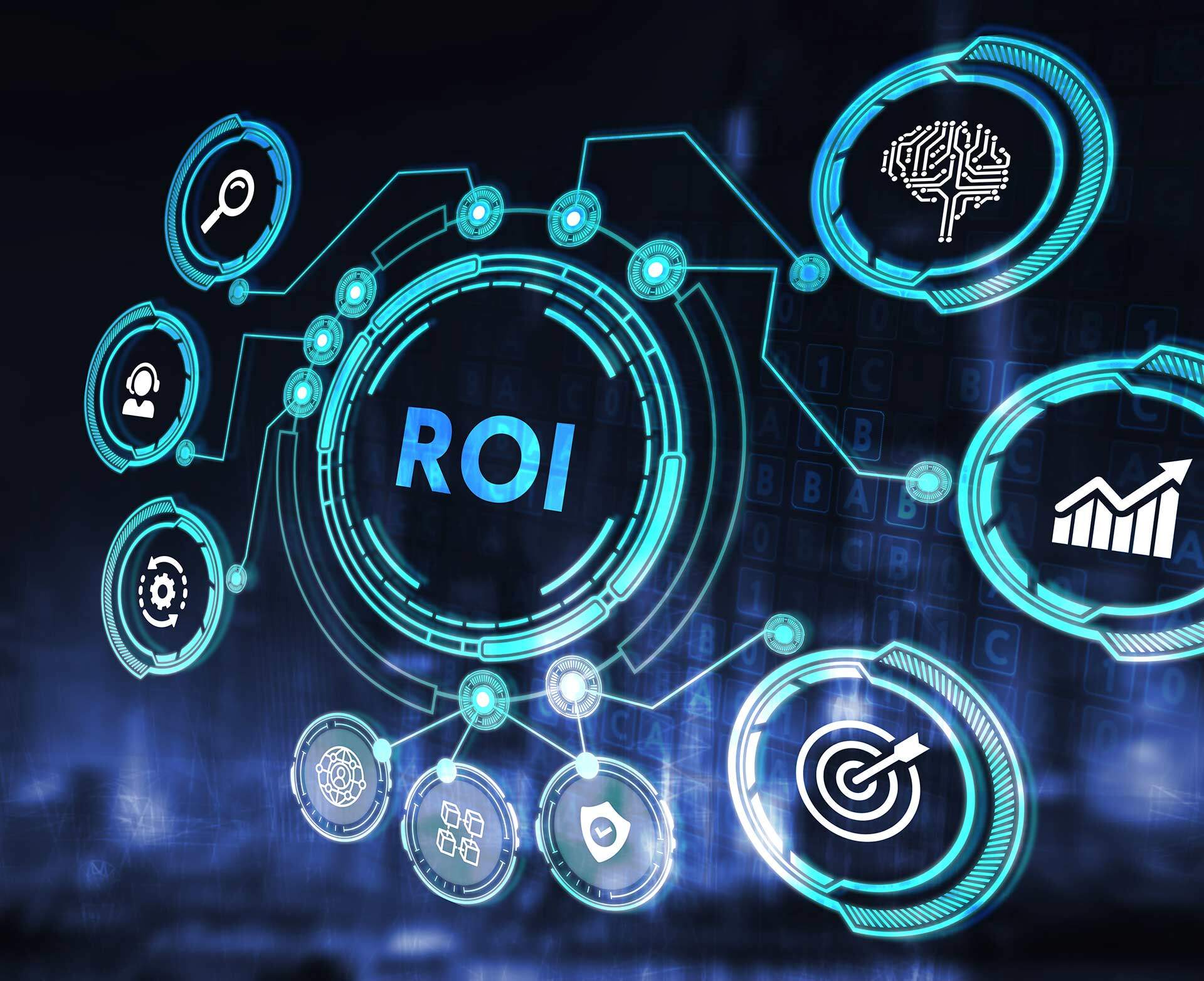
Conclusion
AI solutions for industrial process optimization are both transformative tools and strategic investments. Their value lies in their ability to drive measurable efficiency, predictive insights, and adaptive capabilities across diverse operations.
Choosing the right solution requires a clear alignment between business objectives and technology capabilities, paired with an implementation plan that considers long-term scalability.
With the right approach, AI can seamlessly integrate into existing workflows, delivering sustained improvements and competitive advantages in an increasingly data-driven field.
To unlock the full potential of AI in your operations, choosing the right partner is essential.
With capabilities like production optimization, predictive maintenance, and demand forecasting, Pinja’s AI and machine learning solutions provide end-to-end solutions designed to enhance efficiency and reduce costs.
Our tools also address critical needs such as supply chain transparency, sustainability reporting, and personalized customer experiences, offering both immediate and long-term value.
To explore how Pinja can support your specific goals, connect with our team today for tailored guidance and solutions.
What are the 4 main problems AI can solve?
-
Operational inefficiencies through process automation and optimization.
-
Inaccurate forecasting by analyzing data for demand prediction.
-
High error rates by detecting and preventing quality deviations.
-
Resource mismanagement through improved allocation and supply chain transparency.
What are the 4 types of AI systems?
-
Reactive Machines: Perform specific tasks without memory or learning capabilities.
-
Limited Memory: Learn from past data to make decisions.
-
Theory of Mind: Understand emotions and social interactions (emerging).
-
Self-Aware AI: Hypothetical systems with consciousness and self-awareness.
What are AI solutions?
AI solutions are technologies designed to automate tasks, optimize processes, provide decision-making insights, and improve efficiency. They include predictive maintenance, demand forecasting, workflow automation, and supply chain management tools tailored to specific business needs.
What can AI do for a business?
AI enhances efficiency, reduces operational costs, improves decision-making through analytics, optimizes resource allocation, personalizes customer experiences, ensures quality control, and streamlines compliance with regulatory standards.
Can AI solve an optimization problem?
Yes, AI solves optimization problems by analyzing data to identify inefficiencies and recommend optimal solutions. Examples include improving production schedules, minimizing energy consumption, and optimizing supply chain operations.