Learning analytics refers to the process of collecting, measuring and analyzing information about learners. Learning analytics requires learning data, which can be collected from various sources, such as institutions’ own study registers and learning systems, as well as from external sources such as Statistics Finland and the IT Center for Science. The uses of learning analytics vary, but one of the most common is to monitor the progress of learning.
Learning analytics is at its best descriptive, explanatory and proactiveDeveloping learning analytics is a long-term and multi-dimensional task, but fortunately also a rewarding one. By carefully laying the groundwork for analytics and setting targets at a realistic but steady level, more and more doors will open up over time for the versatile use of data. To ensure that the data can be used for the intended purpose, the structure of the data and the consistency of the records are key – right from the start.
Learning analytics can be divided into three levels: descriptive, explanatory, and proactive. The easiest of these is the descriptive layer, which in practice visualizes existing data for easier review. A relatively small amount of data is sufficient to implement the descriptive level.
A more advanced level is the so-called explanatory level, which already requires some actual analytics behind it. For the explanatory level, you need to define measures to explain phenomena, combine data and define certain weighing factors. Thus, the explanatory level requires a deeper understanding of the subject of the analysis in order not to draw the wrong conclusions from the data.
Ideally, all three levels of learning analytics and reporting are applied simultaneously, supporting each other.
The proactive level is the most challenging of the three, but often the most effective. Foresight allows us to influence developments as early as possible, but it requires advanced technology and, in many cases, machine learning applications.
Ideally, all three levels of learning analytics and reporting are applied simultaneously, supporting each other. While analytical work often becomes more complex as it progresses and becomes more detailed, it is worth remembering that the path is typically upward, and that work done in earlier stages is not wasted – on the contrary. Understanding trends that are difficult to discern and require advanced analysis can be thought of as the tip of the iceberg, which cannot be reached without careful and consistent groundwork.
Learning analytics serves many purposes and audiences
Perhaps the most typical use of learning analytics is to monitor and assess the progress in learning. Optimally, learning analytics can be used, for example, in the proactive assessment of support needs. This helps identify situations that require special attention, and learners who would benefit from additional support at an early stage.
Learning analytics is often specifically designed to monitor and support learning, but it can also be used for other purposes. Analytics can also be used to gain insights into the wider processes of learning, the overall context of institutions and curricula, and the management of an institution. When learning analytics is used to manage an educational institution, it is in effect knowledge management. For this purpose, analytics can open up new perspectives for decision-making, support staff management and help target resources. It also has a role to play in supporting the well-being of students and staff alike.
If the perspective is further broadened, learning analytics even has the potential to strengthen the link between training needs and training provision at national level. Data analytics are already in use, and by expanding the use, they could be used, for example, to link education and training provisions with skills requirements in the workplace.
Three tips to get started with learning analytics
1. Make a bold move
Developing learning analytics is a journey that can start on a very small scale. A realistic target, a small dataset and a structured format will get the job started. Over time, both the amount of data and the targets can be increased, but the underlying targets, logics and data collection should be carefully designed from the start.
2. Sufficient time and resources
In learning analytics, the biggest effort is often an internal reflection within the work community on what issues are to be analyzed, why they should be analyzed, and with what priority; as well as the selection of reliable data sources for the analysis. During the implementation phase, the most important thing is to involve key people from the institution and, if necessary, to bring in additional technical expertise from an external partner.
Please note: Although a learning analytics implementation project often involves only a few contacts in the institution, the resulting reports often benefit a wide range of people, from teaching staff to students.
3. The power of cooperation
As in many other areas, there are real synergies in working together on learning analytics and reporting. Many of the laws apply to all schools at the same level, so there is no point in everyone reinventing the same wheel. Ideally, the base can be common, and specific functionalities can be tailored for each institution as needed.
Read more
Blog: Reporting solutions for educational institutions – how to move to genuine knowledge management?
Success story: University of Jyväskylä
Pinja's Business Intelligence services
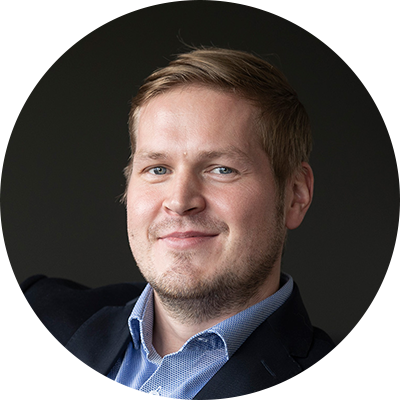
Lasse Mäkinen
I work at Pinja as an Account Manager in the Business Intelligence unit. I help our clients develop knowledge management and modern reporting with Pinja’s BI solutions and services. I spend my leisure time with family and friends, performing a bit of forestry and exercising occasionally – I especially like CrossFit.
Back to the Pinja Blog
Categories
- Pinja Career (71)
- Production development (42)
- Software development (42)
- Business Intelligence (40)
- Digital business (30)
- Circular economy and natural resources (27)
- Sustainability (27)
- Ecommerce (22)
- ICT services (21)
- Maintenance development (21)
- Digital society (20)
- Industrial digitalization (20)
- Supply chain management (19)
- ERP (18)
- Forest industry ERP (13)
- Industrial innovation (11)
- Health and welfare technology (9)
- Artificial intelligence and machine learning (5)
- Lean (4)