The use of AI in predicting and managing business has been discussed for a long time, but so far it has been more talk than action. We are now in a transformational phase, where machine learning is increasingly generating high quality implementations for business use. The use of machine learning, which started from fulfilling the needs of industry, is suitable for almost any sector, and projects based on it can be implemented like any other IT project, as long as the relevant business data is collected in a sufficient quantity and quality.
Any kind of data can be used to make predictions, as long as there is enough of them. In industry, the condition of equipment can be monitored and unforeseen costs avoided, for example by monitoring abnormal resonance on vibration sensors, which indicates the need for equipment maintenance. Sales forecasts, on the other hand, can track the effects of weather or unemployment on demand, in addition to the typical seasonal variations.
Where do you start when machine learning is involved in reporting?
Machine learning projects start by focusing on what you want to achieve and study or predict. The quickest way to get started is to have a preliminary understanding of the factors that influence the predictive data. The best way to do this is to bring together business insight and expertise in data analytics and statistical methods, and to take a deeper look at what kind of data are needed and whether it is currently of sufficient quality.
For a machine learning project to be successful, the client needs to have collected a sufficient amount of data relevant to the business and the issue that is investigated. It is also important that the data are complete and stored in an easily accessible format, for example in a data warehouse. You can get started without a data warehouse, but then you have to start by completing and validating the data set, which takes time and resources. At the very least, the ongoing maintenance and development of machine learning models requires data to be processed in a data warehouse, so the project is more painless if the data warehouse is implemented from the very beginning of the project. A project for our client Fresh Servant, a food company, used a pre-built data warehouse, which made it easy to combine the data.
Machine learning models are tested with an expert
Once enough data have been collected, the extraction process begins. Potential gaps in the data are filled and then the data are split into teaching data and testing data. The testing data are used to find the machine learning models that work best in the given situation. This step requires the in-depth knowledge of a data analyst or data architect. You don’t have to create the models yourself; there is a vast library of models available that can be applied. The choice of models is a trial-and-error process, where the best model is found by testing different options. Usually, one or two models are selected, and the suitable parameters are set for them. Once the models have been selected, the model is tested against live data to ensure that the predictions are correct.
In order to draw the right conclusions from the data, it is important to invest in continuous monitoring. All the forecasts produced and the actual values are captured and analyzed: how accurate are the forecasts, where are the differences and how should the models be improved? Automatic reports can be created to monitor the models, showing their success rate. If, for any reason, the performance of the models deteriorates, they can be reviewed again.
Automated reports to improve forecast accuracy
Automated reports show the accuracy of forecasts, and warn of performance degradation, but a data scientist is needed when models need to be trained to be more accurate. Despite its name, machine learning does not magically learn by itself, but requires external monitoring and guidance. Models need to evolve to reflect changes in business and the environment. The data we process varies, and therefore the models must adapt and change with them. Parameters may need to be modified or completely new parameters added to make the forecasts more accurate. Values that differ from those used in the training phase may emerge. If a model is built to work for a certain range of values, for example 0–100, and the actual values exceed 100, it is assessed whether the model will work for the deviating values or whether it needs to be retrained. It may also turn out that the model does not work as expected for some subset. Identifying these situations and correcting models accordingly requires an understanding of statistics and the ability to mine data.
To make machine learning serve the business in the best way, the client must commit to monitoring and further development together with the model developer. Continuous monitoring is essential to ensure that machine learning can deliver accurate predictions and tangible benefits for the client. Through systematic work machine learning can significantly improve the quality and cost-effectiveness of business operations.
Machine learning helped Fresh Servant reduce waste and improve efficiency
Pinja implemented a project for Fresh Servant, the market leader in consumer packaged salads, where machine learning is used to improve demand forecasting. Very soon after starting the project, the forecasts proved to be more accurate than those produced manually and by mathematical calculation. The project tested different methods and selected those that worked best for Fresh Servant’s customer groups and products. Machine learning-base forecasting now minimizes manual work, but also allows for the addition and use of human input as needed. As a result of the cooperation, the production is already more efficient, reducing waste and improving cost-effectiveness.
However, efforts are being made to further improve the accuracy of the forecasts, for example by identifying more factors explaining demand and incorporating them into the forecasting model. With Pinja’s help, Fresh Servant has the opportunity to apply machine learning to other areas of its business in the future.
Want to hear more?
To learn more about how Pinja can help your business develop its BI insight, please visit our Business Intelligence website. There you will find our knowledge management tutorials, client stories, and service descriptions.
Read more:
Success story: Fresh Servant
Pinja’s Business Intelligence solutions
Blog: A summary on why a data warehouse is such an important part of knowledge management
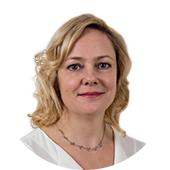
Hanna Salonen
I am a knowledge utilization professional with twenty years of experience in the IT industry and knowledge-based management. The most important thing for me is that the goal set together with the customer is realized, the solution is long-lasting and easy and pleasant to use. My free time is spent e.g. with family, versatile exercise and nature.
Back to the Pinja Blog
Categories
- Pinja Career (71)
- Production development (43)
- Software development (43)
- Business Intelligence (42)
- Digital business (30)
- Sustainability (29)
- Circular economy and natural resources (27)
- Ecommerce (23)
- ICT services (22)
- Maintenance development (22)
- Supply chain management (21)
- Digital society (20)
- Industrial digitalization (20)
- ERP (18)
- Forest industry ERP (14)
- Industrial innovation (11)
- Health and welfare technology (9)
- Artificial intelligence and machine learning (7)
- Lean (4)
- eudr (1)